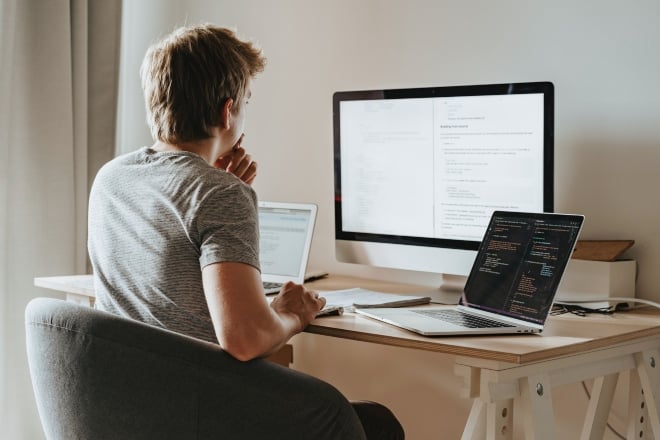
Pexels / olia danilevich
How do you deal with the types of errors in A/B Testing? Experimental error and common error are things that we’ll get to in a bit, too. First, you have to understand the purpose of A/B testing.
A/B Testing helps determine which versions of your content get better traffic and, therefore, better conversion. Often, businesses make A/B Testing mistakes that cost them a lot of time, money, and effort.
Now, let’s begin.
Contents
What are the Types of Errors in A/B Testing
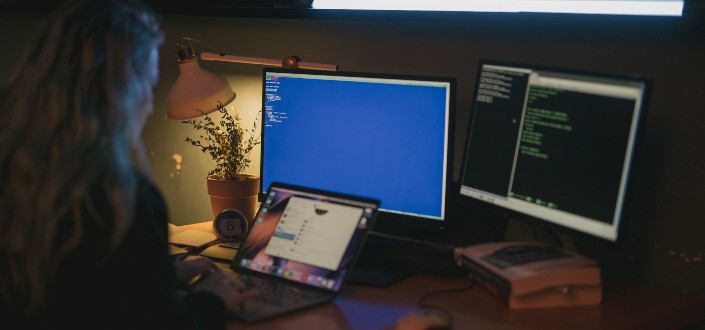
Pexels / cottonbro
As a proprietor, or at least the one handling a company’s digital marketing, it’s important to know what works in promoting your business. One way to determine which factors help boost your web pages’ traffic and possibly conversion and profits is by executing A/B Testing.
Statistics is the main indicator of the different probabilities of A/B Testing. However, not all results might be accurate or precise; this is where the Types of Errors in A/B Testing come in.
Two Types of Errors
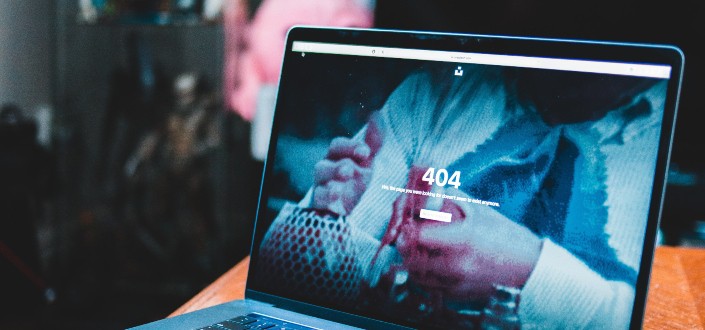
Unsplash / Erik Mclean
It’s worth noting that there are other types of errors to be wary of in any controlled experiment–not just in A/B testing or any split or multivariate tests. For example, getting a systematic error or a random error may be caused by a flawed test design. Syntax errors and logical errors, on the other hand, can be caused by mistakes in labels, values, and other factors in the initial test design.
However, in this post, we’ll focus on the Types of Errors in A/B Testing: Type 1 Error and Type 2 Error. We’ll also discuss their possible causes, risks, fixes, and ways to avoid those errors.
Here are the two types of error in A/B testing:
Type 1 Error
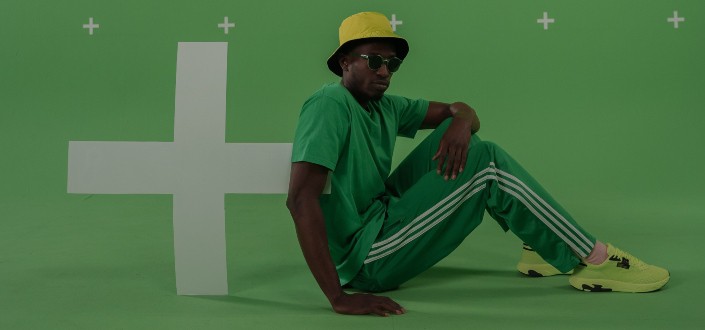
Pexels / cottonbro
Definition:
Type I Errors are more commonly known as false positives. Also called Alpha (α) error, a Type I Error may present better than the variant B or C or so on, or vice versa. This type of error leads you to deploy this “better performing” version only to see a temporary positive outcome.
Causes
In carrying out A/B Testing, critical metrics should be met before concluding a test result. When you commit a Type 1 error, the chances are that there is either a miscalculation or an untimely conclusion. In many cases, a Type I Error may be the outcome of the premature conclusion of the tests.
Risk of Having Type I Error
Ultimately, arriving at a conclusion that leads to low-performing content on your landing page will negatively impact the goals you’ve set for the growth of your business. Whether your plan is hitting a set number of clicks per month or increase in conversion in terms of sales, account sign-ups, and clients, committing a Type I Error can deter the growth you’ve been working on.
How To Fix Type I error
Once you’ve determined which parts of your landing page perform better, you may deploy new changes accordingly, depending on your set factors and metrics. For example, let’s say Version A of your CTA encourages more clicks on your website, then it’s best to retain that rather than persisting on Version B or C that does not perform as well.
How to Avoid Type I error
Completely eliminating the possibility of committing a Type 1 error in A/B Testing is impossible to do. However, some ways might help minimize the chances of making the error on your Test. One of which is minimizing the significance level of your Test. You can do so by running your A/B Test for a longer period (as long as you can afford it) to garner a good sample size-giving you a more accurate and credible result for your conclusion.
Type II Error
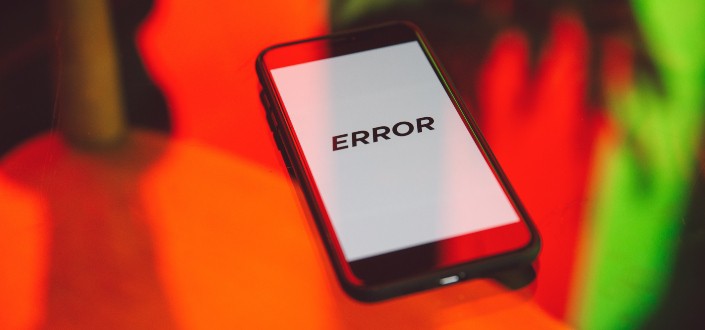
Pexels / Polina Zimmerman
Definition
On the other hand, type 2 Errors or false negatives refer to unsuccessful or inconclusive A/B Testing. Also called Beta (β) errors, this type of result happens when the null hypothesis appears to be true and appears more favorable than your alternative hypothesis. Having a false negative error adversely affects the outcome of the test as well as the decision.
Causes
In A/B Testing, there might be factors that might seem to have a menial effect on the desired outcome/s. As such, Beta (β) errors often result from low statistical power.
Risk of Having Type II error
Similar to the risk of Type I Errors from occurring, several factors may come into the picture. Arriving at this conclusion can lead to discouragement and invalidation of the goals set prior to the experiment or testing.
How To Fix Type II Error
There is no “particular fix” to having a Type 2 Error apart from executing multiple tests but adjusting the factors and metrics before commencing your measurement tests on your AB Testing tool.
How to avoid Type II error
Minimizing a Type 2 Error from occurring all comes down to probability and increasing the statistical power of the test. Increasing the sample size for your test can help avoid Beta (β) errors.
Significance of Running An A/B Test in Improving Your Conversion Rate Optimization
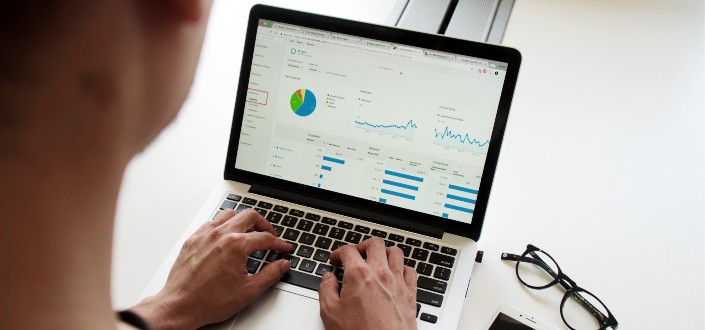
Unsplash / Campaign Creators
Working on your means to achieve conversion optimization for your landing pages is crucial for running a business. However, your experiments and A/BTests might be for naught with the wrong process. And so, making necessary changes prior to testing is essential as this can push your conversions in the right and progressive direction.
There’s no such thing as failed testing, so if your experiment does not meet your goals, take note of the factors that might affect your page’s performance.
- Does your landing page need high-quality copies?
- Does it need stark images or content to invite more clicks and new users?
- Does it present a better user experience or cater to user behavior?
- Do you need a better-performing CTA to get a visitor to subscribe to your service?
Consider the goals you want to achieve in your testing. A high conversion rate will translate to the type of content you put out on your landing page. All of these things are involved in the AB Testing process.
Frequently Asked Questions
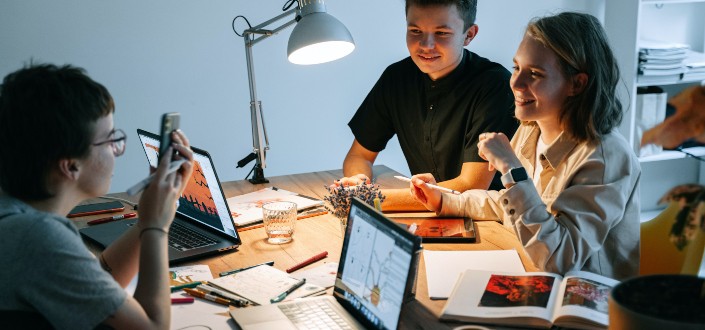
Pexels / Ivan Samkov
Have more questions in mind? We’ll answer the most-asked questions on AB testing work in this section.
Which error is more serious, type 1 or type 2?
In general, getting a Type I error, where you get a false-positive result, is more serious than getting a Type 2 error. However, there are also times when Type 2 errors pose more danger to your experiment. In short, it’s on a case-to-case basis when it comes to AB Testing statistics.
Why do type 1 and type 2 errors not happen simultaneously?
As mentioned before, Type 1 errors have a significant impact on your test’s statistical power. The statistical power, on the other hand, has an inverse relationship with getting a Type 2 error. Therefore, they cannot occur at the same time.
Can you avoid errors?
Avoiding these types of errors is impossible; however, you can reduce the chances of getting them on your hypothesis testing. Reducing or increasing the sample size is one way to go to avoid getting a measurement error in your hypothesis test.
How bad are hypothesis errors?
A/B Testing involves time, effort, and money. And so, getting errors on your experiments, fundamentally, costs a lot. So it’s crucial that you try to avoid these errors and the wrong observation at all costs, especially if you plan to run Google ads or a Facebook ad on your page.
More Interesting Articles To Explore
Want to enhance your writing, add more traffic to your landing pages, and overall improve your marketing strategies for your marketing campaign? You don’t need a degree in Data Science to achieve these things. Here are some interesting and helpful articles to guide you.
- Here is the simplest guide to writing better post titles to invite more users to read your articles.
- Deal with copy anxiety (for your email campaign and other copies) the best way you can with this helpful guide.
- On marketing and drug trials, learn the best practices to reach your target audience.
In Conclusion
A/B Testing is a powerful, straightforward experiment that shows which multiple variations of your landing page perform better or are the more optimized variant. Before conducting an experiment, it’s important that you accept the probability of getting the types of errors in A/B Testing. So whether you encounter any experimental errors or common errors when reading your results, you’re already prepared on what step to do next.